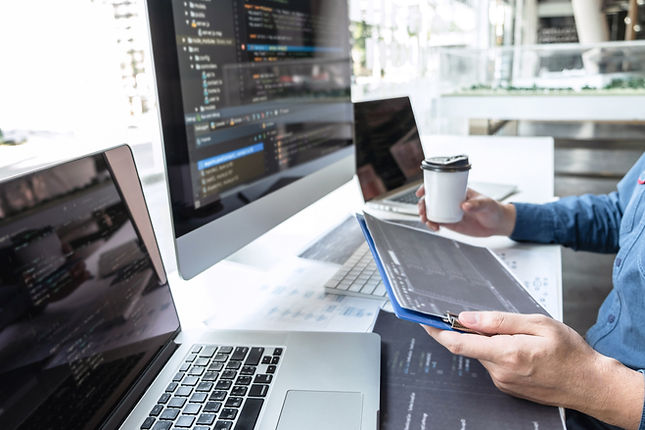
Research Projects
Below, are summarized the projects linked to the Research Grant #2018/15597-6 - Young Investigators Grants - Phase 2.
Application and investigation of unsupervised learning methods in retrieval and classification tasks
Grant number: 2018/15597-6
Support Opportunities: Research Grants - Young Investigators Grants - Phase 2
Duration: May 01, 2019 - July 31, 2024
Field of knowledge: Physical Sciences and Mathematics - Computer Science
Principal Investigator: Daniel Carlos Guimarães Pedronette
Grantee: Daniel Carlos Guimarães Pedronette
Host Institution: Instituto de Geociências e Ciências Exatas (IGCE). Universidade Estadual Paulista (UNESP). Campus de Rio Claro. Rio Claro , SP, Brazil
Associated researchers: Denis Henrique Pinheiro Salvadeo ; Fabricio Aparecido Breve ; João Paulo Papa ; Jurandy Gomes de Almeida Junior ; Ricardo da Silva Torres
​
Abstract
Unsupervised Learning methods have been established as a solution to increase the effectiveness of content-based searches without requiring user intervention. These methods exploit contextual relationship among images, usually encoded in the distance/similarity information of the collections.This research project intends to investigate the application of such methods in new and diversified domains. Unsupervised learning methods reevaluate the similarity between the elements of the collection and can be used as a pre-processing step in classification tasks. In addition, initial results indicate that the methods can be applied in general multimedia and multimodal retrieval scenarios, considering audio and video.Therefore, the central objective of the proposed project is to deepen such investigation, expanding the domains of application of unsupervised learning. methods. (AU)
​
Investigation of Image Noise Reduction based on Unsupervised Learning
Grant number: 2022/14448-2
Support Type: Scholarships in Brazil - Scientific Initiation
Duration: January 01, 2023 - December 31, 2023
Field of knowledge: Physical Sciences and Mathematics - Computer Science
Principal researcher: Daniel Carlos Guimarães Pedronette
Grantee: Murilo Magiolo Geraldini
​
Home Institution: Instituto de Geociências e Ciências Exatas (IGCE). Universidade Estadual Paulista (UNESP). Campus de Rio Claro. Rio Claro , SP, Brazil
​
Abstract
Noise consists of a degradation inherent in the acquisition process, in the most diverse types of imaging. In medical imaging, for example, this may impair or hamper image analysis by the radiologist. In addition, in the case of imaging systems employing ionizing radiation, such as Computed Tomography (CT), Mammography, or Mammary Tomosynthesis, where the search for dose reduction should be somewhat constant according to the ALARA principle (As Low As Reasonably Achievable ), reducing dose leads to increased noise. Thus, considering also that noise can affect subsequent processing, such as segmentation or classification of images, evolving noise filtering techniques is of fundamental importance. Relevant techniques of noise filtering in images, such as Non-Local Means (NLM) Block Matching and 3D Filtering (BM3D), are used in a non-local approach, ie, the similarity between regions (patches) of the image (more than between unit pixels) to perform the filtering. In this sense, the choice of the similarity measure and the technique of selection of more similar patches are essential for the adequate performance of these methods. In this work, we intend to investigate the use of unsupervised learning approaches for replacing similarity measures in order to improve the effectiveness of noise-filtering tasks.
Feature selection and aggregation based on rank measures
Grant number: 2022/07349-8
Support Type: Scholarships in Brazil - Master
Duration: August 01, 2022 - April 30, 2024
Field of knowledge: Physical Sciences and Mathematics - Computer Science
Principal researcher: Daniel Carlos Guimarães Pedronette
Grantee: Ademir Moreno Junior
​
Home Institution: Instituto de Geociências e Ciências Exatas (IGCE). Universidade Estadual Paulista (UNESP). Campus de Rio Claro. Rio Claro , SP, Brazil
Abstract
Despite the significant recent advances in feature extraction methods based on deep learning approaches, effectively retrieving images still remains a challenge in various scenarios. Such complexity is mainly associated with the diverse aspects involved in visual perception, which usually can not be encoded by a single feature. Given the myriad of available features, several fusion approaches have been investigated aiming at producing more effective retrieval results. Despite the success of fusion approaches, another crucial task consists in defining what features to combine. Selecting features (or rankers) capable of producing an effective retrieval result consists of a difficult task, especially in unsupervised scenarios, where no information about the effectiveness of the feature is available. This project aims to address this problem by investigating an unsupervised selection framework based on ranking information. The proposed methodology aims to investigate the use of recently proposed rank correlation measures for analyzing complementary information and recent effectiveness estimation measures to identify the most effective combinations. (AU)
Times series retrieval through unsupervised learning
Grant number: 2022/01359-1
Support Type: Scholarships in Brazil - Master
Duration: April 01, 2022 - March 31, 2024
Field of knowledge: Physical Sciences and Mathematics - Computer Science
Principal researcher: Daniel Carlos Guimarães Pedronette
Grantee: Bionda Rozin
​
Home Institution: Instituto de Geociências e Ciências Exatas (IGCE). Universidade Estadual Paulista (UNESP). Campus de Rio Claro. Rio Claro , SP, Brazil
Abstract
Many applications in science, e-commerce, and surveillance monitoring rely on recording sensor information in regular time intervals. As a consequence, time-series data has been growing tremendously. This flood of data requires data management for fast storage and access. Therefore, similarity search on time series data is a typical requirement for such databases. Besides the time series modeling, the similarity measure adopted for comparing different times series also plays an important role, directly affecting the quality of retrieval results. Traditional solutions often perform only pairwise analysis, that is, the similarity measure considers only the information given by the pairs of time series being compared. In this scenario, the relationships among objects modeled by the time series are not considered. Therefore, the intrinsic structure of the collection is ignored. In the context of this project, we intend to consider: (i) Use of unsupervised learning methods to increase the effectiveness of time series retrieval tasks by considering contextual information and relationships among data items; (ii) Comparative study considering different unsupervised learning methods for time series retrieval. (AU)
Rank-based unsupervised learning through deep learning in diverse domains
Grant number: 2020/02183-9
Support Type: Scholarships in Brazil - Post-Doctorate
Duration: April 01, 2020 - March 31, 2024
Field of knowledge: Physical Sciences and Mathematics - Computer Science
Principal researcher: Daniel Carlos Guimarães Pedronette
Grantee: Vanessa Helena Pereira Ferrero
​
Home Institution: Instituto de Geociências e Ciências Exatas (IGCE). Universidade Estadual Paulista (UNESP). Campus de Rio Claro. Rio Claro , SP, Brazil
​
Abstract
Ranking-based unsupervised learning methods have been established as a solution to increase the effectiveness of content-based searches without requiring user intervention. These methods exploit contextual relationships among images, usually encoded in the distance/similarity information of the collections. Concomitantly, strategies based on deep learning have assumed a prominent position in the most diverse machine learning tasks. Thus, this research project aims to combine these two lines of research, investigating new unsupervised learning methods that are based on ranking information and deep learning. In addition, it is also intended to investigate other retrieval and classification scenarios, expanding the domains of application of unsupervised learning methods. (AU)
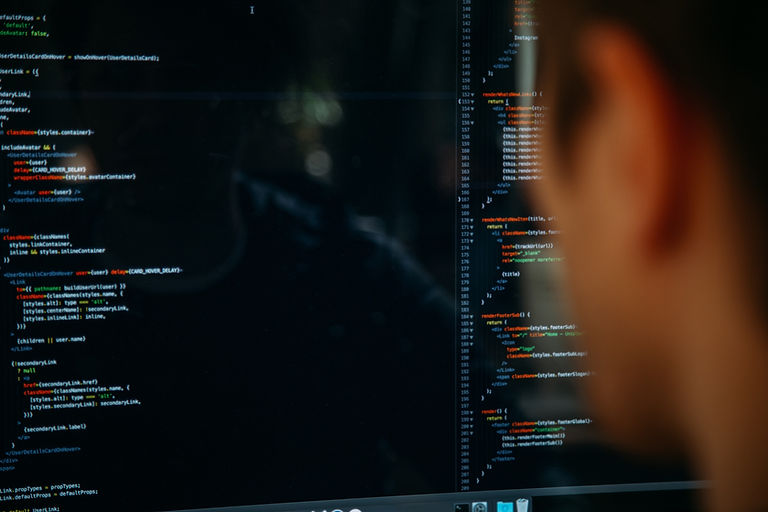